Transformer (Attention Is All You Need) 구현하기 (2/3)
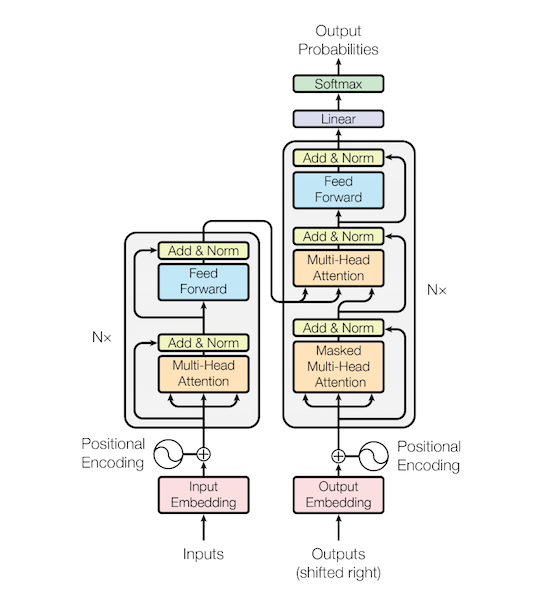
Transformer (Attention Is All You Need) 구현하기 (1/3)에서 포스팅된 내용을 기반으로 Encoder, Decoder 및 Transformer 모델 전체를 설명 하겠습니다.
이 포스트는 Transformer 모델 구현에 대한 설명 입니다. 논문에 대한 내용은 Attention Is All You Need 논문을 참고 하거나 다른 블로그를 참고 하세요.
미리 확인해야할 포스트
- Sentencepiece를 활용해 Vocab 만들기
- Naver 영화리뷰 감정분석 데이터 전처리 하기
- Transformer (Attention Is All You Need) 구현하기 (1/3)
1. Config
Transformer (Attention Is All You Need) 구현하기 (1/3)의 코드처럼 Transformer 모델에는 많은 설정이 필요합니다. 이 설정을 json 형태로 저장을 하고 이를 읽어서 처리하는 간단한 클래스 입니다.
1
2
3
4
5
6
7
8
9
10
""" configuration json을 읽어들이는 class """
class Config(dict):
__getattr__ = dict.__getitem__
__setattr__ = dict.__setitem__
@classmethod
def load(cls, file):
with open(file, 'r') as f:
config = json.loads(f.read())
return Config(config)
작은 리소스에서도 동작 가능하도록 여러 파라미터를 작게 설정 했습니다. 가지고 계신 GPU가 여유가 있다면 파라미터를 키우면 더 좋은 결과를 확인 할 수 있을 겁니다. 기본 파라미터는 config.json을 참고 하세요.
1
2
3
4
5
6
7
8
9
10
11
12
13
14
15
config = Config({
"n_enc_vocab": len(vocab),
"n_dec_vocab": len(vocab),
"n_enc_seq": 256,
"n_dec_seq": 256,
"n_layer": 6,
"d_hidn": 256,
"i_pad": 0,
"d_ff": 1024,
"n_head": 4,
"d_head": 64,
"dropout": 0.1,
"layer_norm_epsilon": 1e-12
})
print(config)
아래와 같은 파라미터 정보를 확인 할 수 있습니다.
1
{'n_enc_vocab': 8007, 'n_dec_vocab': 8007, 'n_enc_seq': 256, 'n_dec_seq': 256, 'n_layer': 6, 'd_hidn': 256, 'i_pad': 0, 'd_ff': 1024, 'n_head': 4, 'd_head': 64, 'dropout': 0.1, 'layer_norm_epsilon': 1e-12}
2. Common Class
Transformer (Attention Is All You Need) 구현하기 (1/3)에서 설명한 ‘Position Embedding’, ‘Multi-Head Attention’, ‘Feeed Forward’등의 코드 입니다.
Position Encoding
Position Embedding의 초기 값을 구하는 함수 입니다.
- 각 position별 hidden index별 angle값을 구합니다. (줄: 8)
- hidden 짝수 index의 angel값의 sin값을 합니다. (줄: 9)
- hidden 홀수 index의 angel값의 cos값을 합니다. (줄: 10)
1
2
3
4
5
6
7
8
9
10
11
12
""" sinusoid position encoding """
def get_sinusoid_encoding_table(n_seq, d_hidn):
def cal_angle(position, i_hidn):
return position / np.power(10000, 2 * (i_hidn // 2) / d_hidn)
def get_posi_angle_vec(position):
return [cal_angle(position, i_hidn) for i_hidn in range(d_hidn)]
sinusoid_table = np.array([get_posi_angle_vec(i_seq) for i_seq in range(n_seq)])
sinusoid_table[:, 0::2] = np.sin(sinusoid_table[:, 0::2]) # even index sin
sinusoid_table[:, 1::2] = np.cos(sinusoid_table[:, 1::2]) # odd index cos
return sinusoid_table
Attention Pad Mask
Attention을 구할 때 Padding 부분을 제외하기 위한 Mask를 구하는 함수 입니다.
- K의 값 중에 Pad인 부분을 True로 변경 합니다. (나머지는 False) (줄: 5)
- 구해진 값의 크기를 Q-len, K-len 되도록 변경 합니다. (줄: 6)
1
2
3
4
5
6
7
""" attention pad mask """
def get_attn_pad_mask(seq_q, seq_k, i_pad):
batch_size, len_q = seq_q.size()
batch_size, len_k = seq_k.size()
pad_attn_mask = seq_k.data.eq(i_pad)
pad_attn_mask= pad_attn_mask.unsqueeze(1).expand(batch_size, len_q, len_k)
return pad_attn_mask
Attention Decoder Mask
Decoder의 ‘Masked Multi Head Attention’에서 사용할 Mask를 구하는 함수 입니다. 현재단어와 이전단어는 볼 수 있고 다음단어는 볼 수 없도록 Masking 합니다.
- 모든 값이 1인 Q-len, K-len 테이블을 생성 합니다. (줄: 3)
- 대각선을 기준으로 아래쪽을 0으로 만듭니다. (줄: 4)
1
2
3
4
5
""" attention decoder mask """
def get_attn_decoder_mask(seq):
subsequent_mask = torch.ones_like(seq).unsqueeze(-1).expand(seq.size(0), seq.size(1), seq.size(1))
subsequent_mask = subsequent_mask.triu(diagonal=1) # upper triangular part of a matrix(2-D)
return subsequent_mask
Scaled Dot Product Attention
Scaled Dot Product Attention을 구하는 클래스 입니다.
- Q * K.transpose를 구합니다. (줄: 11)
- K-dimension에 루트를 취한 값으로 나줘 줍니다. (줄: 12)
- Mask를 적용 합니다. (줄: 13)
- Softmax를 취해 각 단어의 가중치 확률분포 attn_prob를 구합니다. (줄: 15)
- attn_prob * V를 구합니다. 구한 값은 Q에 대한 V의 가중치 합 벡터입니다.
1
2
3
4
5
6
7
8
9
10
11
12
13
14
15
16
17
18
19
20
""" scale dot product attention """
class ScaledDotProductAttention(nn.Module):
def __init__(self, config):
super().__init__()
self.config = config
self.dropout = nn.Dropout(config.dropout)
self.scale = 1 / (self.config.d_head ** 0.5)
def forward(self, Q, K, V, attn_mask):
# (bs, n_head, n_q_seq, n_k_seq)
scores = torch.matmul(Q, K.transpose(-1, -2))
scores = scores.mul_(self.scale)
scores.masked_fill_(attn_mask, -1e9)
# (bs, n_head, n_q_seq, n_k_seq)
attn_prob = nn.Softmax(dim=-1)(scores)
attn_prob = self.dropout(attn_prob)
# (bs, n_head, n_q_seq, d_v)
context = torch.matmul(attn_prob, V)
# (bs, n_head, n_q_seq, d_v), (bs, n_head, n_q_seq, n_v_seq)
return context, attn_prob
Multi-Head Attention
Multi-Head Attention을 구하는 클래스 입니다.
- Q * W_Q를 한 후 multi-head로 나눕니다. (줄: 17)
- K * W_K를 한 후 multi-head로 나눕니다. (줄: 19)
- V * W_V를 한 후 multi-head로 나눕니다. (줄: 21)
- ScaledDotProductAttention 클래스를 이용해 각 head별 Attention을 구합니다. (줄: 27)
- 여러 개의 head를 1개로 합칩니다. (줄: 29)
- Linear를 취해 최종 Multi-Head Attention값을 구합니다. (줄: 31)
1
2
3
4
5
6
7
8
9
10
11
12
13
14
15
16
17
18
19
20
21
22
23
24
25
26
27
28
29
30
31
32
33
34
""" multi head attention """
class MultiHeadAttention(nn.Module):
def __init__(self, config):
super().__init__()
self.config = config
self.W_Q = nn.Linear(self.config.d_hidn, self.config.n_head * self.config.d_head)
self.W_K = nn.Linear(self.config.d_hidn, self.config.n_head * self.config.d_head)
self.W_V = nn.Linear(self.config.d_hidn, self.config.n_head * self.config.d_head)
self.scaled_dot_attn = ScaledDotProductAttention(self.config)
self.linear = nn.Linear(self.config.n_head * self.config.d_head, self.config.d_hidn)
self.dropout = nn.Dropout(config.dropout)
def forward(self, Q, K, V, attn_mask):
batch_size = Q.size(0)
# (bs, n_head, n_q_seq, d_head)
q_s = self.W_Q(Q).view(batch_size, -1, self.config.n_head, self.config.d_head).transpose(1,2)
# (bs, n_head, n_k_seq, d_head)
k_s = self.W_K(K).view(batch_size, -1, self.config.n_head, self.config.d_head).transpose(1,2)
# (bs, n_head, n_v_seq, d_head)
v_s = self.W_V(V).view(batch_size, -1, self.config.n_head, self.config.d_head).transpose(1,2)
# (bs, n_head, n_q_seq, n_k_seq)
attn_mask = attn_mask.unsqueeze(1).repeat(1, self.config.n_head, 1, 1)
# (bs, n_head, n_q_seq, d_head), (bs, n_head, n_q_seq, n_k_seq)
context, attn_prob = self.scaled_dot_attn(q_s, k_s, v_s, attn_mask)
# (bs, n_head, n_q_seq, h_head * d_head)
context = context.transpose(1, 2).contiguous().view(batch_size, -1, self.config.n_head * self.config.d_head)
# (bs, n_head, n_q_seq, e_embd)
output = self.linear(context)
output = self.dropout(output)
# (bs, n_q_seq, d_hidn), (bs, n_head, n_q_seq, n_k_seq)
return output, attn_prob
FeeedForward
FeeedForward를 처리하는 클래스 입니다.
- Linear를 실행하여 shape을 d_ff(hidden * 4) 크기로 키웁니다. (줄: 14)
- activation 함수(relu or gelu)를 실행합니다. (줄: 15)
- Linear를 실행하여 shape을 hidden 크기로 줄입니다. (줄: 17)
1
2
3
4
5
6
7
8
9
10
11
12
13
14
15
16
17
18
19
20
""" feed forward """
class PoswiseFeedForwardNet(nn.Module):
def __init__(self, config):
super().__init__()
self.config = config
self.conv1 = nn.Conv1d(in_channels=self.config.d_hidn, out_channels=self.config.d_ff, kernel_size=1)
self.conv2 = nn.Conv1d(in_channels=self.config.d_ff, out_channels=self.config.d_hidn, kernel_size=1)
self.active = F.gelu
self.dropout = nn.Dropout(config.dropout)
def forward(self, inputs):
# (bs, d_ff, n_seq)
output = self.conv1(inputs.transpose(1, 2))
output = self.active(output)
# (bs, n_seq, d_hidn)
output = self.conv2(output).transpose(1, 2)
output = self.dropout(output)
# (bs, n_seq, d_hidn)
return output
3. Encoder
Encoder Layer
Encoder에서 루프를 돌며 처리 할 수 있도록 EncoderLayer를 정의하고 여러 개 만들어서 실행 합니다.
- Multi-Head Attention을 수행합니다. (줄: 14)
Q, K, V 모두 동일한 값을 사용하는 self-attention 입니다. - 1번의 결과와 input(residual)을 더한 후 LayerNorm을 실행 합니다. (줄: 15)
- 2번의 결과를 입력으로 Feed Forward를 실행 합니다. (줄: 17)
- 3번의 결과와 2번의 결과(residual)을 더한 후 LayerNorm을 실행 합니다. (줄: 18)
1
2
3
4
5
6
7
8
9
10
11
12
13
14
15
16
17
18
19
20
""" encoder layer """
class EncoderLayer(nn.Module):
def __init__(self, config):
super().__init__()
self.config = config
self.self_attn = MultiHeadAttention(self.config)
self.layer_norm1 = nn.LayerNorm(self.config.d_hidn, eps=self.config.layer_norm_epsilon)
self.pos_ffn = PoswiseFeedForwardNet(self.config)
self.layer_norm2 = nn.LayerNorm(self.config.d_hidn, eps=self.config.layer_norm_epsilon)
def forward(self, inputs, attn_mask):
# (bs, n_enc_seq, d_hidn), (bs, n_head, n_enc_seq, n_enc_seq)
att_outputs, attn_prob = self.self_attn(inputs, inputs, inputs, attn_mask)
att_outputs = self.layer_norm1(inputs + att_outputs)
# (bs, n_enc_seq, d_hidn)
ffn_outputs = self.pos_ffn(att_outputs)
ffn_outputs = self.layer_norm2(ffn_outputs + att_outputs)
# (bs, n_enc_seq, d_hidn), (bs, n_head, n_enc_seq, n_enc_seq)
return ffn_outputs, attn_prob
Encoder
Encoder 클래스 입니다.
- 입력에 대한 Position 값을 구합니다. (줄: 14~16)
- Input Embedding과 Position Embedding을 구한 후 더합니다. (줄: 19)
- 입력에 대한 attention pad mask를 구합니다. (줄: 22)
- for 루프를 돌며 각 layer를 실행합니다. (줄: 27)
layer의 입력은 이전 layer의 출력 값 입니다.
1
2
3
4
5
6
7
8
9
10
11
12
13
14
15
16
17
18
19
20
21
22
23
24
25
26
27
28
29
30
""" encoder """
class Encoder(nn.Module):
def __init__(self, config):
super().__init__()
self.config = config
self.enc_emb = nn.Embedding(self.config.n_enc_vocab, self.config.d_hidn)
sinusoid_table = torch.FloatTensor(get_sinusoid_encoding_table(self.config.n_enc_seq + 1, self.config.d_hidn))
self.pos_emb = nn.Embedding.from_pretrained(sinusoid_table, freeze=True)
self.layers = nn.ModuleList([EncoderLayer(self.config) for _ in range(self.config.n_layer)])
def forward(self, inputs):
positions = torch.arange(inputs.size(1), device=inputs.device, dtype=inputs.dtype).expand(inputs.size(0), inputs.size(1)).contiguous() + 1
pos_mask = inputs.eq(self.config.i_pad)
positions.masked_fill_(pos_mask, 0)
# (bs, n_enc_seq, d_hidn)
outputs = self.enc_emb(inputs) + self.pos_emb(positions)
# (bs, n_enc_seq, n_enc_seq)
attn_mask = get_attn_pad_mask(inputs, inputs, self.config.i_pad)
attn_probs = []
for layer in self.layers:
# (bs, n_enc_seq, d_hidn), (bs, n_head, n_enc_seq, n_enc_seq)
outputs, attn_prob = layer(outputs, attn_mask)
attn_probs.append(attn_prob)
# (bs, n_enc_seq, d_hidn), [(bs, n_head, n_enc_seq, n_enc_seq)]
return outputs, attn_probs
4. Decoder
Decoder Layer
Decoder에서 루프를 돌며 처리 할 수 있도록 DecoderLayer를 정의하고 여러 개 만들어서 실행 합니다.
- Multi-Head Attention을 수행합니다. (줄: 16)
Q, K, V 모두 동일한 값을 사용하는 self-attention 입니다. - 1번의 결과와 input(residual)을 더한 후 LayerNorm을 실행 합니다. (줄: 17)
- Encoder-Decoder Multi-Head Attention을 수행합니다. (줄: 19)
Q: 2번의 결과
K, V: Encoder 결과 - 3번의 결과와 2번의 결과(residual)을 더한 후 LayerNorm을 실행 합니다. (줄: 20)
- 4번의 결과를 입력으로 Feed Forward를 실행 합니다. (줄: 22)
- 5번의 결과와 4번의 결과(residual)을 더한 후 LayerNorm을 실행 합니다. (줄: 23)
1
2
3
4
5
6
7
8
9
10
11
12
13
14
15
16
17
18
19
20
21
22
23
24
25
""" decoder layer """
class DecoderLayer(nn.Module):
def __init__(self, config):
super().__init__()
self.config = config
self.self_attn = MultiHeadAttention(self.config)
self.layer_norm1 = nn.LayerNorm(self.config.d_hidn, eps=self.config.layer_norm_epsilon)
self.dec_enc_attn = MultiHeadAttention(self.config)
self.layer_norm2 = nn.LayerNorm(self.config.d_hidn, eps=self.config.layer_norm_epsilon)
self.pos_ffn = PoswiseFeedForwardNet(self.config)
self.layer_norm3 = nn.LayerNorm(self.config.d_hidn, eps=self.config.layer_norm_epsilon)
def forward(self, dec_inputs, enc_outputs, self_attn_mask, dec_enc_attn_mask):
# (bs, n_dec_seq, d_hidn), (bs, n_head, n_dec_seq, n_dec_seq)
self_att_outputs, self_attn_prob = self.self_attn(dec_inputs, dec_inputs, dec_inputs, self_attn_mask)
self_att_outputs = self.layer_norm1(dec_inputs + self_att_outputs)
# (bs, n_dec_seq, d_hidn), (bs, n_head, n_dec_seq, n_enc_seq)
dec_enc_att_outputs, dec_enc_attn_prob = self.dec_enc_attn(self_att_outputs, enc_outputs, enc_outputs, dec_enc_attn_mask)
dec_enc_att_outputs = self.layer_norm2(self_att_outputs + dec_enc_att_outputs)
# (bs, n_dec_seq, d_hidn)
ffn_outputs = self.pos_ffn(dec_enc_att_outputs)
ffn_outputs = self.layer_norm3(dec_enc_att_outputs + ffn_outputs)
# (bs, n_dec_seq, d_hidn), (bs, n_head, n_dec_seq, n_dec_seq), (bs, n_head, n_dec_seq, n_enc_seq)
return ffn_outputs, self_attn_prob, dec_enc_attn_prob
Decoder
Decoder 클래스 입니다.
- 입력에 대한 Position 값을 구합니다. (줄: 14~16)
- Input Embedding과 Position Embedding을 구한 후 더합니다. (줄: 19)
- 입력에 대한 attention pad mask를 구합니다. (줄: 22)
- 입력에 대한 decoder attention mask를 구합니다. (줄: 24)
- attention pad mask와 decoder attention mask 중 1곳이라도 mask되어 있는 부분인 mask 되도록 attention mask를 구합니다. (줄: 26)
- Q(decoder input), K(encoder output)에 대한 attention mask를 구합니다. (줄: 28)
- for 루프를 돌며 각 layer를 실행합니다. (줄: 27)
layer의 입력은 이전 layer의 출력 값 입니다.
1
2
3
4
5
6
7
8
9
10
11
12
13
14
15
16
17
18
19
20
21
22
23
24
25
26
27
28
29
30
31
32
33
34
35
36
37
""" decoder """
class Decoder(nn.Module):
def __init__(self, config):
super().__init__()
self.config = config
self.dec_emb = nn.Embedding(self.config.n_dec_vocab, self.config.d_hidn)
sinusoid_table = torch.FloatTensor(get_sinusoid_encoding_table(self.config.n_dec_seq + 1, self.config.d_hidn))
self.pos_emb = nn.Embedding.from_pretrained(sinusoid_table, freeze=True)
self.layers = nn.ModuleList([DecoderLayer(self.config) for _ in range(self.config.n_layer)])
def forward(self, dec_inputs, enc_inputs, enc_outputs):
positions = torch.arange(dec_inputs.size(1), device=dec_inputs.device, dtype=dec_inputs.dtype).expand(dec_inputs.size(0), dec_inputs.size(1)).contiguous() + 1
pos_mask = dec_inputs.eq(self.config.i_pad)
positions.masked_fill_(pos_mask, 0)
# (bs, n_dec_seq, d_hidn)
dec_outputs = self.dec_emb(dec_inputs) + self.pos_emb(positions)
# (bs, n_dec_seq, n_dec_seq)
dec_attn_pad_mask = get_attn_pad_mask(dec_inputs, dec_inputs, self.config.i_pad)
# (bs, n_dec_seq, n_dec_seq)
dec_attn_decoder_mask = get_attn_decoder_mask(dec_inputs)
# (bs, n_dec_seq, n_dec_seq)
dec_self_attn_mask = torch.gt((dec_attn_pad_mask + dec_attn_decoder_mask), 0)
# (bs, n_dec_seq, n_enc_seq)
dec_enc_attn_mask = get_attn_pad_mask(dec_inputs, enc_inputs, self.config.i_pad)
self_attn_probs, dec_enc_attn_probs = [], []
for layer in self.layers:
# (bs, n_dec_seq, d_hidn), (bs, n_dec_seq, n_dec_seq), (bs, n_dec_seq, n_enc_seq)
dec_outputs, self_attn_prob, dec_enc_attn_prob = layer(dec_outputs, enc_outputs, dec_self_attn_mask, dec_enc_attn_mask)
self_attn_probs.append(self_attn_prob)
dec_enc_attn_probs.append(dec_enc_attn_prob)
# (bs, n_dec_seq, d_hidn), [(bs, n_dec_seq, n_dec_seq)], [(bs, n_dec_seq, n_enc_seq)]S
return dec_outputs, self_attn_probs, dec_enc_attn_probs
5. Transformer
Transformer 클래스 입니다.
- Encoder Input을 입력으로 Encoder를 실행합니다. (줄: 12)
- Encoder Output과 Decoder Input을 입력으로 Decoder를 실행합니다. (줄: 14)
1
2
3
4
5
6
7
8
9
10
11
12
13
14
15
16
""" transformer """
class Transformer(nn.Module):
def __init__(self, config):
super().__init__()
self.config = config
self.encoder = Encoder(self.config)
self.decoder = Decoder(self.config)
def forward(self, enc_inputs, dec_inputs):
# (bs, n_enc_seq, d_hidn), [(bs, n_head, n_enc_seq, n_enc_seq)]
enc_outputs, enc_self_attn_probs = self.encoder(enc_inputs)
# (bs, n_seq, d_hidn), [(bs, n_head, n_dec_seq, n_dec_seq)], [(bs, n_head, n_dec_seq, n_enc_seq)]
dec_outputs, dec_self_attn_probs, dec_enc_attn_probs = self.decoder(dec_inputs, enc_inputs, enc_outputs)
# (bs, n_dec_seq, n_dec_vocab), [(bs, n_head, n_enc_seq, n_enc_seq)], [(bs, n_head, n_dec_seq, n_dec_seq)], [(bs, n_head, n_dec_seq, n_enc_seq)]
return dec_outputs, enc_self_attn_probs, dec_self_attn_probs, dec_enc_attn_probs
6. 참고
다음 포스트 Transformer (Attention Is All You Need) 구현하기 (3/3)에서는 Transformer를 이용해서 ‘Naver 영화리뷰 감정분석’ 과정을 정리 하겠습니다.
자세한 내용은 다음을 참고 하세요.